Based within the Ocean Frontier Institute and Department of Biology at Dalhousie University, our lab conducts quantitative fisheries research across a range of marine and freshwater environments. Led by Aaron MacNeil, our group uses models, theory, and collaboration to solve applied fisheries problems around the world. Much of our research deals with management and conservation of fish and other animals in the face of global warming, from tropical to polar regions.
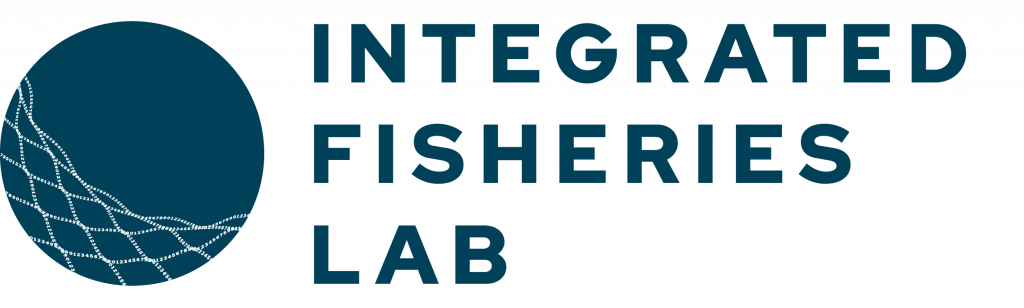